Product information management (PIM) systems centralize data and operations, helping teams work efficiently and maintain consistent product data. AI brings even more value to these solutions, as it reduces many processes to mere seconds.
With AI, you can extract attributes and categorize products, receive recommendations for missing data, and gain insights into inventory. These processes are automated and are a lifesaver for teams working with millions of SKUs (stock-keeping units).
In this post, we focus on how AI powers product information management systems for search and product discovery. Our experts review how AI enhances search relevance, aids in tagging and categorization, and more. Read on to learn how these intelligences and automation in PIM make teams’ work efficient, accurate, and personalized for customers.
What is a Product Information Management (PIM) system
PIM systems are hubs for managing various types of product data, including titles, descriptions, images, videos, and more. In PIM, the data is stored and updated by different teams, and the updates can be accessed and distributed to connected platforms. As a result, any information updates made by your team will be visible to your customers.
That’s a convenient approach. Before PIM systems were introduced, teams relied on spreadsheets and emails to make and align on any changes. PIM systems are a game changer as they help you remove that chaos and streamline product data management with a single source of truth.
In particular, you can use a PIM system to search and optimize product data almost in real-time. This took years of evolution, and now it's possible to search and discover products using AI with greater efficiency.
The evolution of search and discovery in PIM
If we look at the history of PIM, we’ll see that search and discovery have always been essential parts of the purchase process. Here are the critical milestones of PIM:
- 1980s-1990s - manual product data management, rise of mail-order catalogues and bar codes;
- 2000s - rise of PIM solutions, Google influences buying behavior;
- 2010s - PIM integration, rise of omnichannel ecommerce, importance of customer reviews, cloud-based PIM with an accent on security and data redundancy;
- 2010s-2020s - AI for analytics and trend prediction, AR trials, direct-to-consumer sales;
- 2020s - global expansion, ecommerce boom due to the pandemic, emphasis on sustainability, data protection, business norms, and better information management.
In this context, search and discovery have also evolved, starting from basic keyword search techniques and progressing to AI-enhanced functionalities. Keyword search provides teams only with basic finding capabilities. This type of search does not offer fuzzy search or synonym detection, as it is based on static categories.
In contrast, AI-enhanced search provides quick results based on context, even through millions of items. It also provides smart filters, suggestions, and dynamic classification.
AI-powered search: transforming product findability
Traditional keyword-based search often returns incorrect or irrelevant results. As teams shift towards using AI-enhanced PIM search, they can easily find the necessary items or information.
Thanks to advanced data understanding, AI highlights the items based on context, intent, and search history to provide the most relevant results. Here are several essential aspects that make multichannel product findability with AI search in PIM convenient and swift.
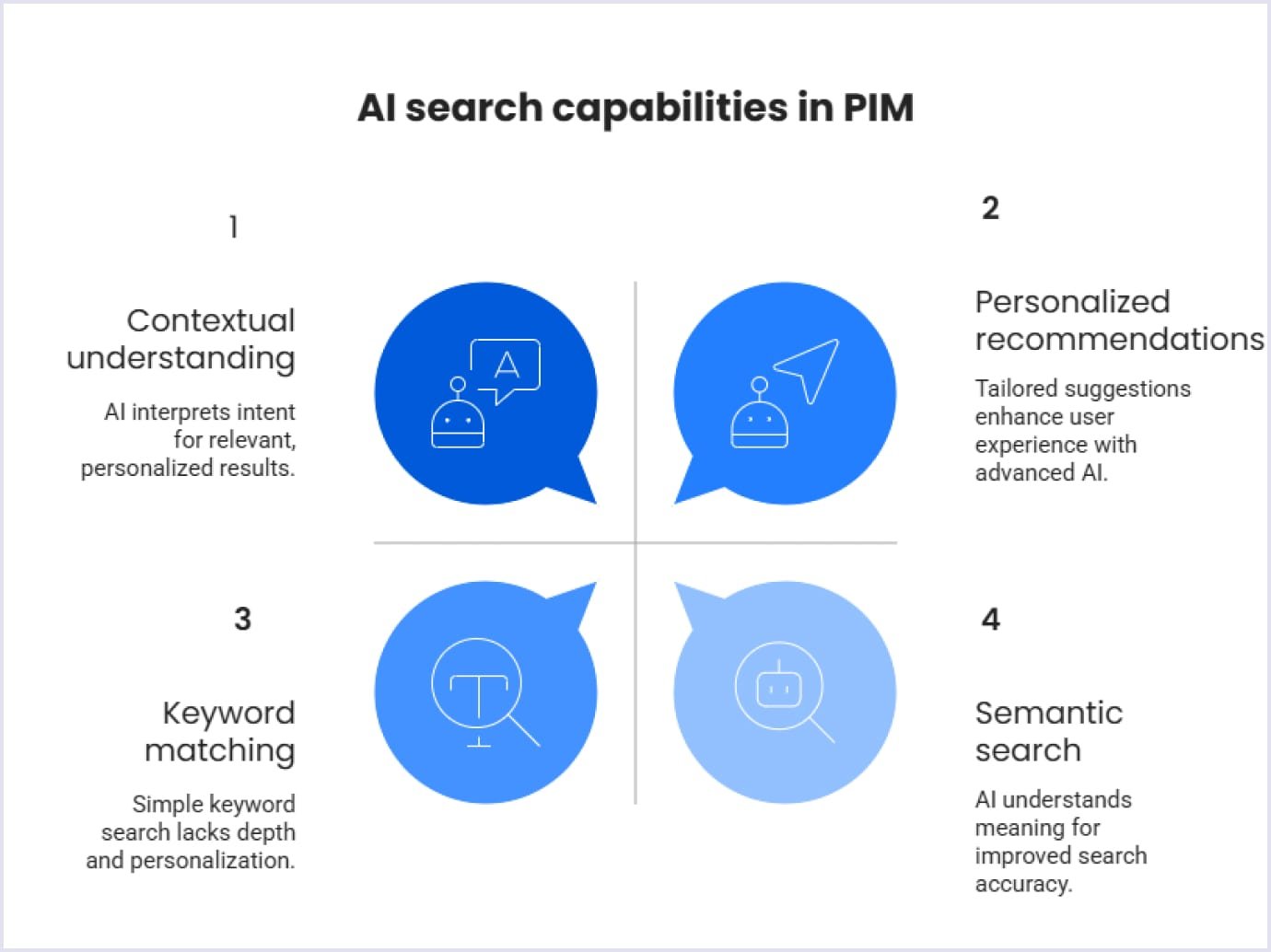
Improved search relevance
With semantic and contextual product recommendations, AI search results are more relevant to user intent compared to traditional term-based findings. AI uses customer search intent analysis to catch the meaning behind a query, not just words. Thus, it provides attribute-based matching, synonyms, variants, fuzzy search in case of typos, and ranking the best contextual fit.
For instance, if you search for “running shoes for winter,” the AI system will return relevant products, such as insulated sneakers tagged for cold weather. Notice that the system will still understand this even without the word “winter” added to the product description.
Read also: Improving Marketplace Search Using Algolia or Elasticsearch
Natural Language Processing (NLP) capabilities
What makes NLP a lifesaver is its versatility and ability to highlight contextually valid items. It accepts input in natural phrases and long-tail queries. NLP models also interpret search queries based on the context, autocorrection, and the ability to support multiple languages.
Thanks to its capabilities, NLP in PIM systems is primarily used for creating and enriching product content. For example, using NLP, AI creates localized descriptions based on key attributes.
Personalized search results
AI learns from past search queries and personalizes results for your team. For example, AI models can learn from team members’ roles and adjust results as needed for personalized recommendations. Also, the AI systems observe how you search, that is, your terms, filters, and recently edited products. It then uses that data to re-rank future search results.
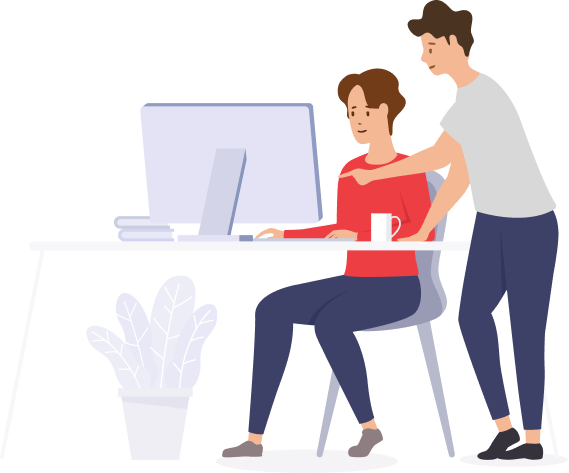
Enhancing internal product discovery through AI in PIM
Product discovery in relation to teams using PIM means finding products to further correct and improve them. Thus, teams use AI-driven product discovery for enrichment, tagging and classification, campaign planning, and quality control.
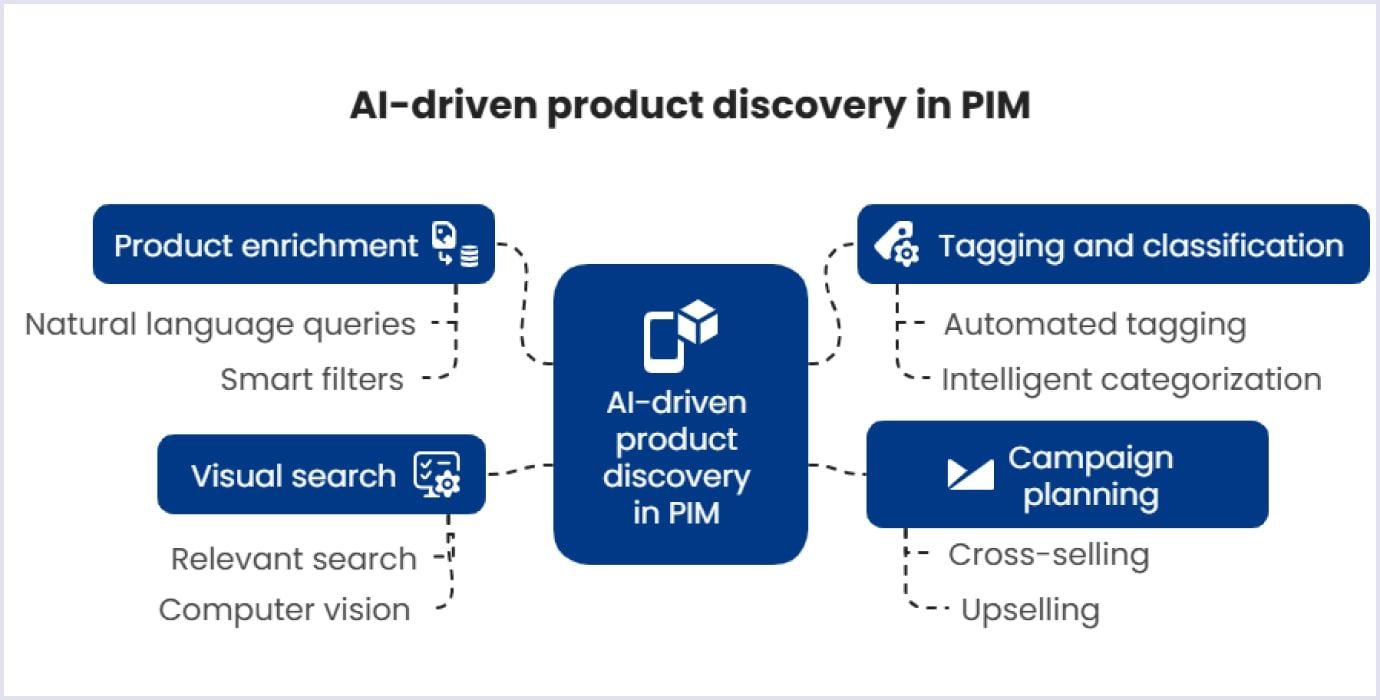
AI helps teams discover, tag, and enrich products faster
These three aspects are essential for modern AI-powered systems used by product managers, merchandisers, content editors, and ecommerce operations. Thanks to AI, teams cooperate and work on changes and updates faster and more accurately, compared to traditional PIM.
In AI-powered PIM, teams can find products based on natural language queries, identify incomplete product descriptions with smart filters, and get prioritized highlights based on team members’ roles. Moreover, AI automates tagging and product enrichment for teams and detects missing data with intelligent product categorization.
Cross-selling and upselling opportunities
While these processes actually happen on the storefront, PIM supports them on the backend with omnichannel product discovery. As AI suggests complementary products, the team can create bundles of products and then enrich and tag them for publication on the website and other digital touchpoints. As a result of comprehensive product data enrichment and product taxonomy automation, teams can launch smart cross-selling and upselling campaigns.
Visual search integration
As product catalogues become larger, visual search can be more convenient than keyword queries. Computer vision technology helps customers discover products, particularly in categories such as fashion, furniture, home decor, automotive, cosmetics, and food.
AI algorithms can find and categorize products based on color, shape, patterns, and materials. They can also suggest visually similar items, even if those characteristics aren’t described in the text, which improves search relevance optimization. Based on this data, AI can identify oversaturated or underrepresented categories, missing sizes or variants, and opportunities to meet customer demand with the right products.
For example, the AI Enrich feature in Bluestone PIM helps you analyze images and extract essential attributes, such as color, size, and style.
Case studies: successful AI integration in PIM systems
INTERSPORT: enhancing team workflow efficiency with UnifAI technology
Being a leader in the sports goods industry in France, Intersport faced challenges in managing product information for its catalog of 50,000 products. The company understood the need to use a PIM system to manage product information, improving team workflow efficiency.
That’s when they called on Akeneo to help them streamline their product information management. Akeneo responded with an AI-powered PIM that utilizes UnifAI technology.
With this AI solution in the PIM, INTERSPORT’s team can classify products with precision, saving 50% on classification time. Moreover, it helped the team to track their budget, sell-outs, and orders.
KLiNGEL: improved business operations with AI-powered PIM system
Europe’s leading fashion brand needed to manage 3 million product SKUs and 90 terabytes of product images. This is where Informatica and its partner, Parsionate, came into play. KLiNGEL evaluated several options and chose Informatica’s Master Data Management (MDM) solution.
Not only does it help KLiNGEL manage their extensive product data, but it also streamlines their business planning with the aid of AI. Thus, the company automates the creation and enrichment of product data. Future plans include automatic classification with semantic data fields, extracting product attributes, and identifying likely matches during imports to prevent duplicates in the product database.
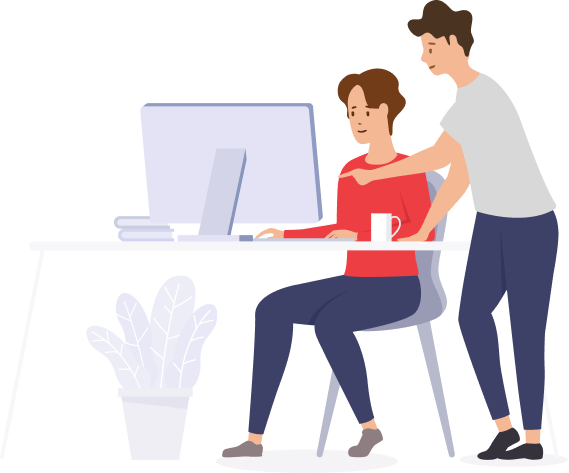
Challenges and considerations in implementing AI in PIM
Data quality and consistency
Adding AI to your PIM requires product data standardization and consistency. It’s not uncommon for data used in PIM to have inconsistent attributes, missing essential components, and historical data from legacy systems.
To improve data quality, use relevant audits and data governance policies. It will establish proper standards for product attribute optimization and taxonomies. Also, it is advisable to use only standardized data for AI to achieve better performance and cross-channel consistency.
Integration with existing systems
If existing systems are not synchronized and do not support modern technologies, it may lead to worsened AI performance. To avoid these integration challenges:
- Choose a PIM system with strong integration capabilities, such as REST API, pre-built connectors, and middleware support;
- Check that specific datasets are clearly held in a particular system;
- Ensure real-time or near-real-time synchronization for product data updates.
Cost and resource allocation
Implementing AI into PIM typically requires the following costs:
- AI-enhanced PIM licenses or add-ons;
- Costs for integration with existing systems;
- Time-intensive data auditing, cleansing, and standardization;
- Teams’ training to help them understand how to use AI;
- Ongoing model optimization.
How can you optimize your costs when implementing AI into PIM? Here are several considerations:
- Start with clear goals to avoid overspending and feature bloat. We recommend you begin with the project discovery phase for a comprehensive business and tech analysis of AI in your PIM;
- Focus on one parameter first, such as product enrichment, and then scale;
- Compare AI search ROI measurement to investment in implementation;
- Allocate the budget not just for technology, but also for training, support, and data governance.
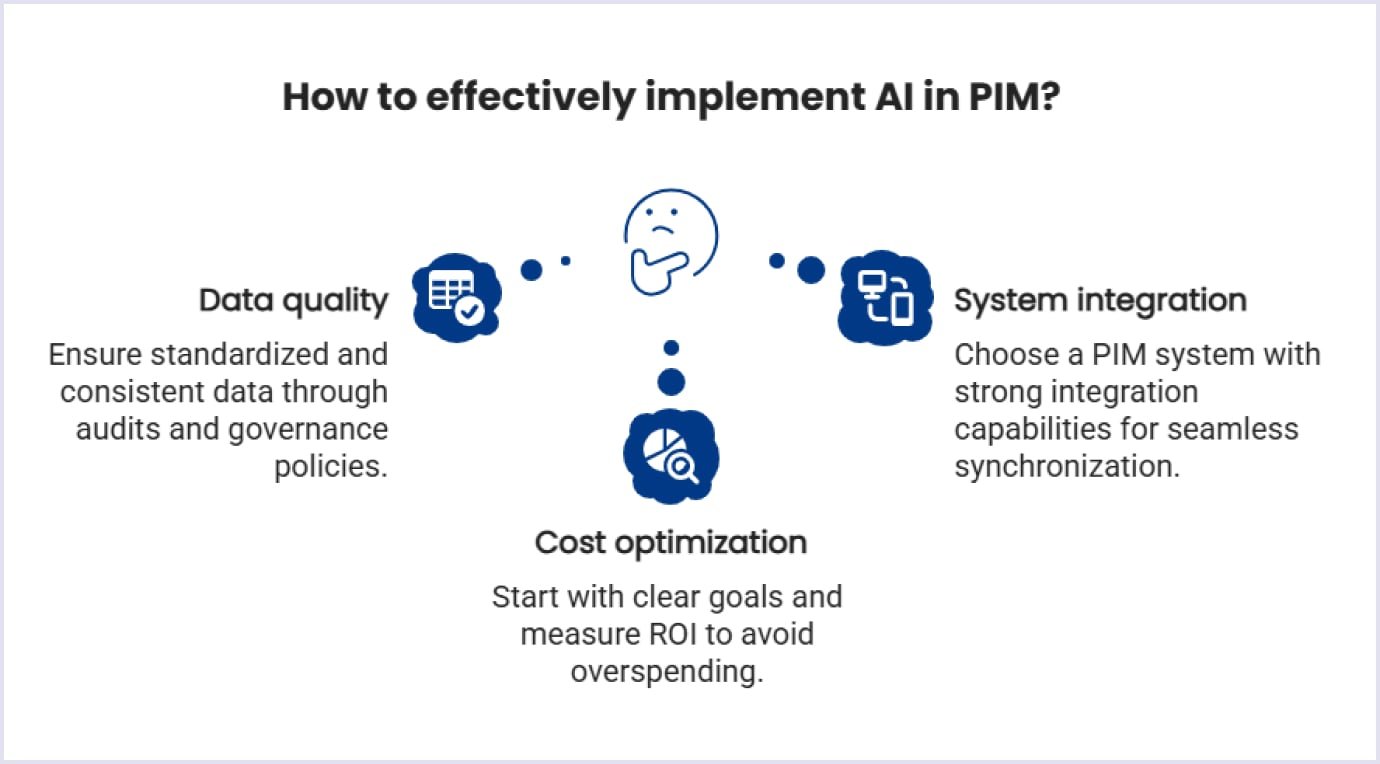
Future trends: the role of AI in PIM moving forward
AI in PIM evolves over time, providing more relevant and precise results in search and product discovery. As for the future, here are several trends that will shape AI in PIM systems:
- Semantic search, Natural Language Processing, and visual product search technology. Searching for products using natural language and visuals is becoming more common due to their ease of use.
- Automated PIM data enrichment and attribute extraction. AI technologies help extract attributes from external sources and populate product descriptions with relevant attributes when they are missing.
- Intelligent product classification and taxonomy management. With AI, teams can classify products based on their attributes and descriptions more quickly and accurately.
- Real-time data validation and quality control. AI-powered PIM systems monitor data for anomalies, inconsistencies, and missing information with product data quality management. This approach ensures accurate information is consistently available across all channels.
- Predictive analytics for demand forecasting. Based on historical sales data and market trends, AI in PIM helps companies to get insights into demand, pricing, and marketing campaigns.
You may also like: AI Pricing: How Much Does AI Cost in 2025?
To conclude
AI in PIM solutions enhances many processes thanks to its ability to handle extensive data, spot patterns, and deliver precision, even when your team works with dozens of products. In this regard, search and product discovery tools help teams find products, identify what needs to be added, and enhance a company's marketing efforts.
If you need a PIM solution for your operations, we can create it for you using our years of experience in custom software development services. We specialize in PIM implementation for startups and established companies, helping them manage complex product data and enrich PIM with AI development services. Feel free to contact us, and our team will provide guidance on development and offer you a quote.